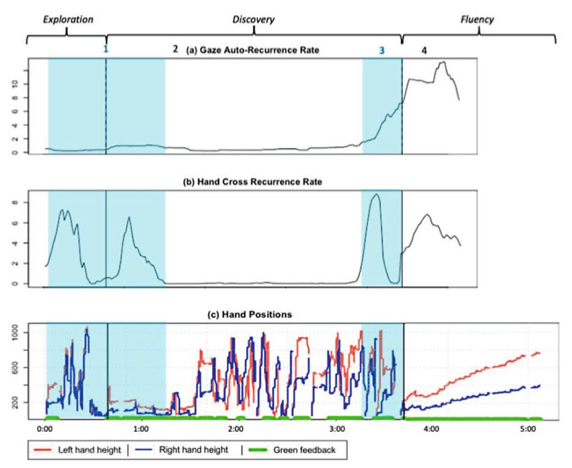
Embodied cognitive science suggests that our bodily activity is part of our cognitive dynamics. I work with multimodal data (including movement and eye tracking, speech, video, electrodermal activity, sensor and touchscreen data) to empirically test this view. I draw in nonlinear methods like Recurrence Quantification Analysis, new to education research, that can capture the complex dynamics of learning data. I apply these methods to study changing coordinations of bodily and ecological resources as learning. For example, I study how shifts in hand movement coordination precede conceptual breakthroughs, and how changing patterns of eye movement reflect new ways of perceiving and consequently, thinking.
Key Publications
Abdu, R., Tancredi, S., Abrahamson, D., & Balasubramaniam, R. (2023). A complex systems outlook on hand-eye coordination in mathematical learning. In M. Schindler, A. Shvarts, & A. Lilienthal. (Eds.), Eye-tracking research in mathematics education [Special issue]. Educational Studies in Mathematics.
Tancredi, S., Abdu, R., Balasubramaniam, R., & Abrahamson, D. (2022). Intermodality in multimodal learning analytics for cognitive theory development: A case from embodied design for mathematics learning. In M. Giannakos, D. Spikol, D. Di Mitri, K. Sharma, X. Ochoa, & R. Hammad (Eds.), Multimodal learning analytics. Springer.
Tancredi, S., Abdu, R., Abrahamson, D., & Balasubramaniam, R. (2021, 2021/04/03/). Modeling nonlinear dynamics of fluency development in an embodied-design mathematics learning environment with Recurrence Quantification Analysis. International Journal of Child-Computer Interaction, 100297. https://doi.org/10.1016/j.ijcci.2021.100297